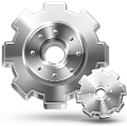
Creative Technology Works LLC
Business Intelligence + Application Development
iAI Search - Find Latent/Hidden Knowledge in your Data!
Real-world data is messy. It often spans media types (e.g. text documents, spreadsheets, PDF files, images, databases), changes constantly, and carries valuable knowledge in ways that is not readily usable. We see the same challenges that emerge from this on a daily basis: We can apply AI retrieval solutions, such as Artificial Intelligence Search - combined with AI models and user friendly custom search applications and dashboards to extract latent and buried knowledge in these vast data stores.
The typical solution pattern for this is a data ingestion, enrichment and exploration model. Each of these brings its own challenges to the table—from large scale change tracking to file format support, and even composition of multiple AI models. Developers can do this today, but it takes a huge amount of effort, requires branching into multiple unrelated domains (from cracking PDFs to handling AI model composition), and distracts from the primary goal. This is where Artificial Intelligence Search comes in.
We can implement Artificial Intelligence Search solutions to find patterns, features, and characteristics in source data, returning structures and textual content that can be used in full-text search solutions.


-
Ingest: pull data from any source - Documents, flat files, images, SQL DB, CosmosDB, PDFs..etc..etc. . For unstructured data the service not only reads the raw data but also supports extracting contents from popular file formats such as PDFs and Office documents. The service supports change detection to keep up with changes through incremental processing, without having to go over the entire data set after initial ingestion.
-
Enrich: use cognitive skills to augment data as it’s ingested using a powerful composition system. The service offers built-in support for OCR (for print and handwritten text), named entity recognition, key phrase extraction, language detection, image analysis with scene description/tagging capabilities, and more. Knowledge created during enrichment often not only augments individual data items, but also connects entities and facts across different items, different stores and even different media types.
-
Explore: the outcome of enrichment is additional knowledge derived from the ingested data combined with the outcome of applying various AI models to it. The original data and all annotations produced during enrichment are put in an Search index—a powerful data store that supports keyword search, dashboarding, custom application intergration and reporting with the ability to handle structured queries in addition to unstructured search, and offers faceted navigation.
Customer Scenarios
Healthcare customers face a similar challenge with clinical data. Large volume of text includes references to general entities (e.g. people’s names) and domain-specific ones (e.g. drug and disease names) that need to be connected and related. Sometimes they also need to combine this with imagery that’s analyzed in well-known ways (e.g. OCR) as well as applying leading-edge methods (e.g. AI-assisted diagnostics).
​
Financial Services space, customers need to handle the challenge of extensive regulation described as large volume of documents, forms produced by their customers, contracts they handle with customers and providers, and more. Generally-applicable natural language processing techniques combined with specialized content understanding models enables them to provide their employees and customers with a global view of their information assets.
​
Oil & Gas companies have teams of geologists and other specialists that need to understand seismic and geologic data. They often have decades of PDFs with pictures of samples over sample sheets full of handwritten field notes. They need to connect places, people (domain experts), events, and navigate all this information to make key decisions.